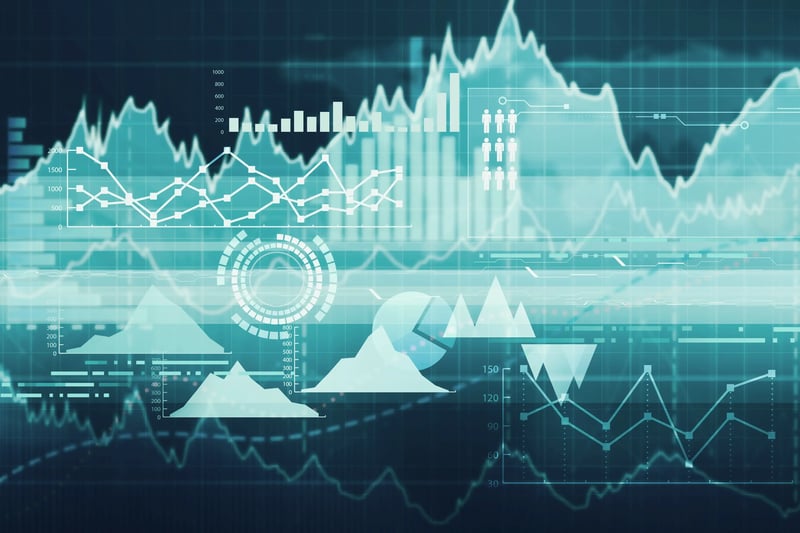
In the complex landscape of oncology research and drug development, precision is paramount. The ability to select appropriate models can significantly impact the success and validity of your research. This is where metadata steps in as your reliable guide for model selection.
Understanding Metadata in Research
Metadata, essentially data about data, plays a crucial role in organizing, describing, and finding datasets. In the context of oncology research, metadata encompasses all the details about experiments, datasets, and results. This includes information on sample types, experimental conditions, data collection methods, and software used. By providing a structured summary of data, metadata helps streamline the process of data retrieval and utilization, for model selection and complex data analysis.
Metadata serves as a roadmap, guiding researchers to the datasets they need, while also providing context and ensuring that data is used appropriately. It’s an essential tool for maintaining consistency and transparency in research. The better structured and more detailed the metadata, the easier it is to manage and interpret large datasets, which are common in oncology.
In oncology research, metadata might include details such as the type of tissue samples collected, the sequencing methods used, and the specific conditions under which experiments were conducted. This ensures that datasets remain comprehensible and reusable, even years after the initial research was completed.
The Importance of Accurate Metadata in Model Selection
Accurate metadata is the backbone of effective model selection. It provides the necessary context to understand datasets fully, ensuring that models are selected based on relevant and precise information. This is particularly important in oncology research, where the accuracy of findings can have significant implications for patient outcomes.
Champions Oncology’s entire database can be explored accessing Lumin, our proprietary integrated software solution that harnesses the power of precisely curated oncology data, computational science, and bioinformatics to help you make data-driven decisions in model selection and data interpretation. Within Lumin you can:
- visualize proprietary genomic, proteomic, and pharmacological profiles from Champions’ patient-derived preclinical models;
- generate analyses on unique multi-omic datasets assembled from over 12,000 patients, including thousands of clinical treatment responses not available in public databases;
- interrogate experimental data and accelerate your oncology research programs.
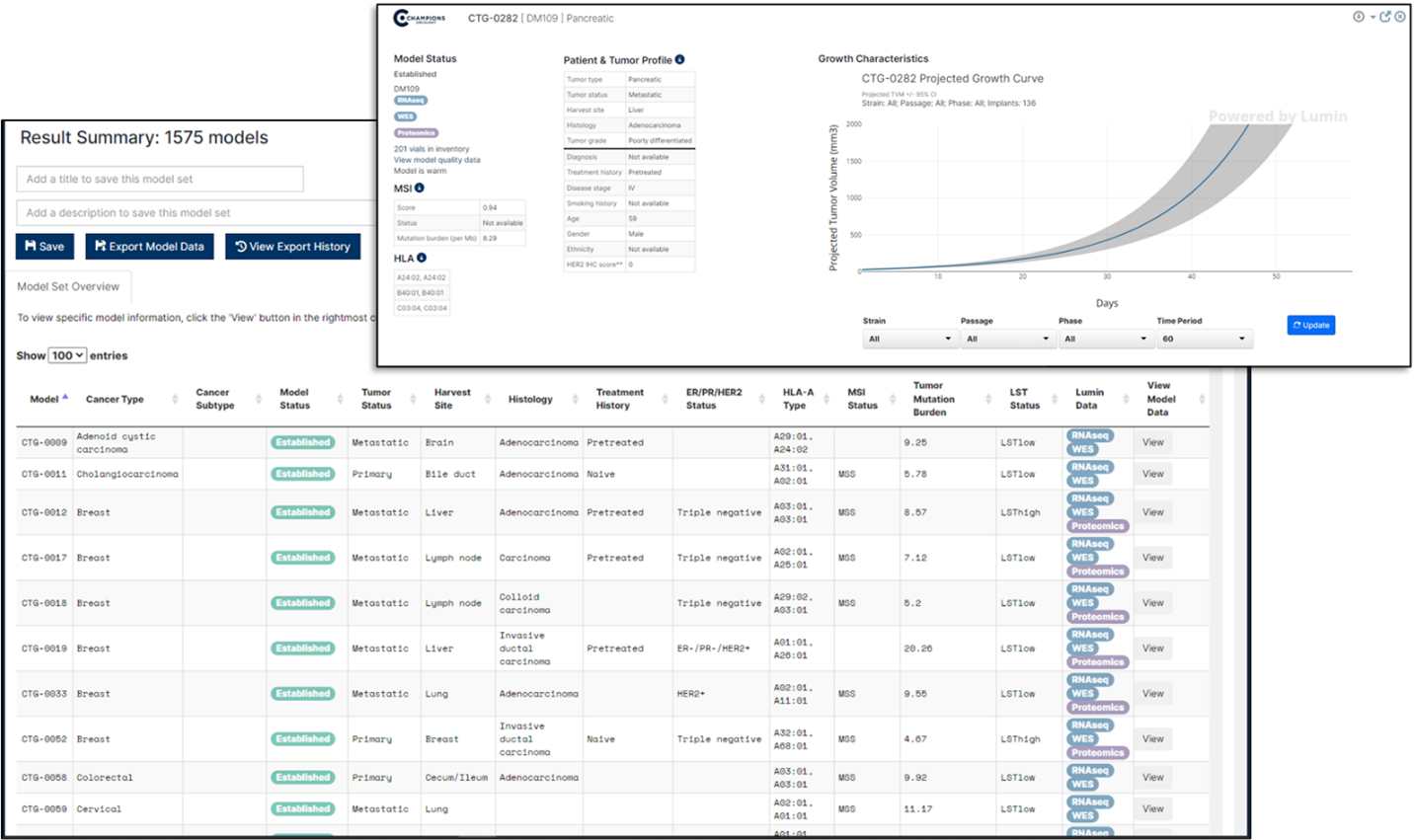
Enhancing Model Selection
With accurate metadata, researchers can filter through vast amounts of data to find the most relevant datasets for their specific needs. For instance, if you're looking for data on a particular cancer type and drug target, well curated metadata can help you quickly identify and access the relevant datasets, saving time and resources.
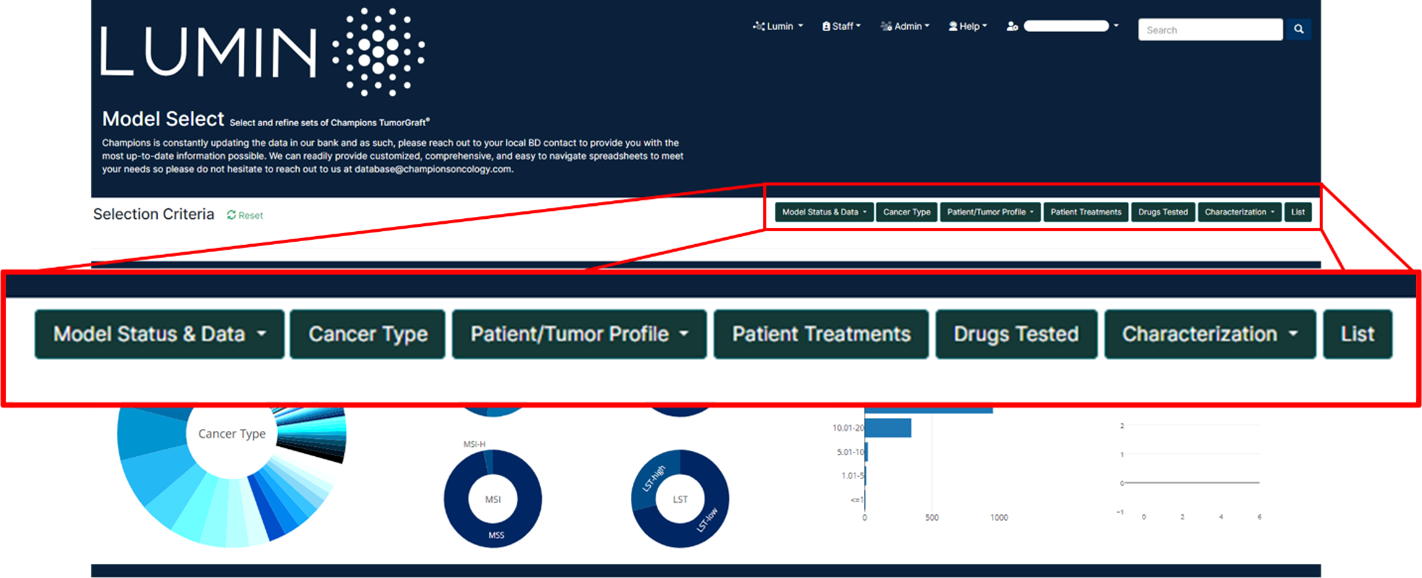
Scientists planning to study the efficacy of a novel targeted therapy developed to treat tumors of a specific type that are resistant to the standard of care for that tumor type could use metadata to select the best set of PDX models to use in their study. Within Lumin, they could filter our bank of tumor models by tumor type first, and then, by drug response profile.
In the example below, we selected all NSCLC PDX models derived from patients who were pre-treated with osimertinib and that did not respond to osimertinib treatment in vivo. This set of models would well represent the target patient population for our drug.
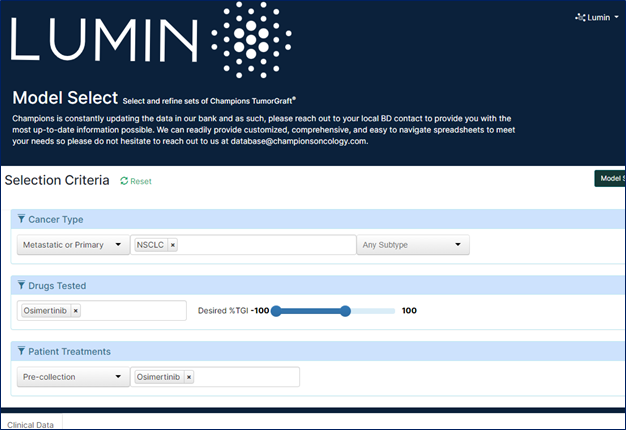
Additionally, scientists could further select models based on gene mutation and RNA and/or protein expression levels of their agent’s target.
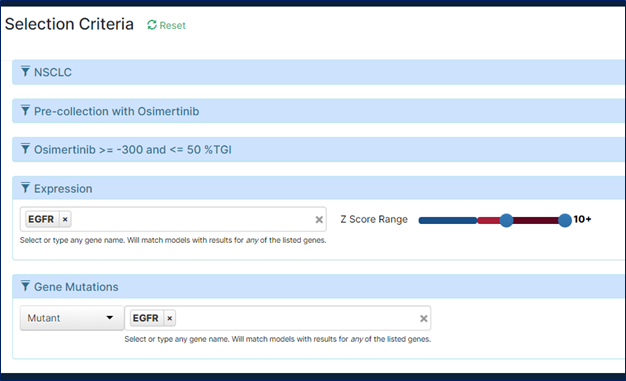
A deeper look into the tumor model metadata, leveraging data analysis and visualization tools, can help fine-tuning model selection further improving the quality of the study results.
Deep characterization and extensive clinical annotation of patient-derived tumor models, allows for a more precise model selection, which translates into a well-designed mouse clinical trial that closely reproduces the clinical setting and patient target population, and ultimately leads to more accurate results and a faster track to clinical experimentation.
Best Practices in Structuring Metadata
To harness the full potential of metadata, it's essential to follow best practices in its structuring and management. Here are some key guidelines for oncology researchers.
Standardized Formats
Using standardized formats for metadata ensures consistency and compatibility across different datasets and platforms. This makes it easier to integrate and compare data from various sources, enhancing the overall quality and reliability of your research.
Detailed Descriptions
Detailed descriptions of the datasets, including information on the origin of the data, the methods used for collection and analysis, and any relevant environmental or experimental conditions are very important. The more detailed your metadata, the more useful it will be for model selection and to bridge with future research.
Regular Updates
Keeping metadata up-to-date to reflect any changes or new findings is paramount. Regular updates ensure that data remains relevant and accurate, supporting the ongoing use and application of any research findings.
Future Trends in Metadata for Research and Biotech
The landscape of metadata is continually evolving, driven by advances in technology and increasing demands for data accuracy and transparency. Here are some future trends that are likely to shape the use of metadata in oncology research.
AI and Machine Learning
Artificial intelligence (AI) and machine learning are set to revolutionize the way we manage and utilize metadata. These technologies can automate the process of metadata generation and analysis, making it faster and more efficient. For example, AI algorithms can analyze large datasets to identify patterns and correlations that might be missed by human researchers.
Integration with Big Data
As the volume of data in oncology research continues to grow, there will be an increasing need for robust metadata systems that can handle big data. Integrating metadata with big data platforms will enhance the ability to manage, analyze, and interpret vast amounts of information, leading to more accurate and comprehensive research outcomes.
Metadata is an invaluable tool for oncology researchers, providing the context and structure needed to manage complex datasets effectively. By following best practices in model selection, you can enhance the accuracy and efficiency of your research, leading to better outcomes and discoveries. The incorporation of metadata into your research processes not only supports better model selection and data integrity but also fosters collaboration and innovation.
Ready to advance your oncology pipeline with faster model selection and AI-powered data analytics & visualizations? Sign up for our free trial of Lumin and discover how we can help you streamline your model selection and accelerate your discoveries.
